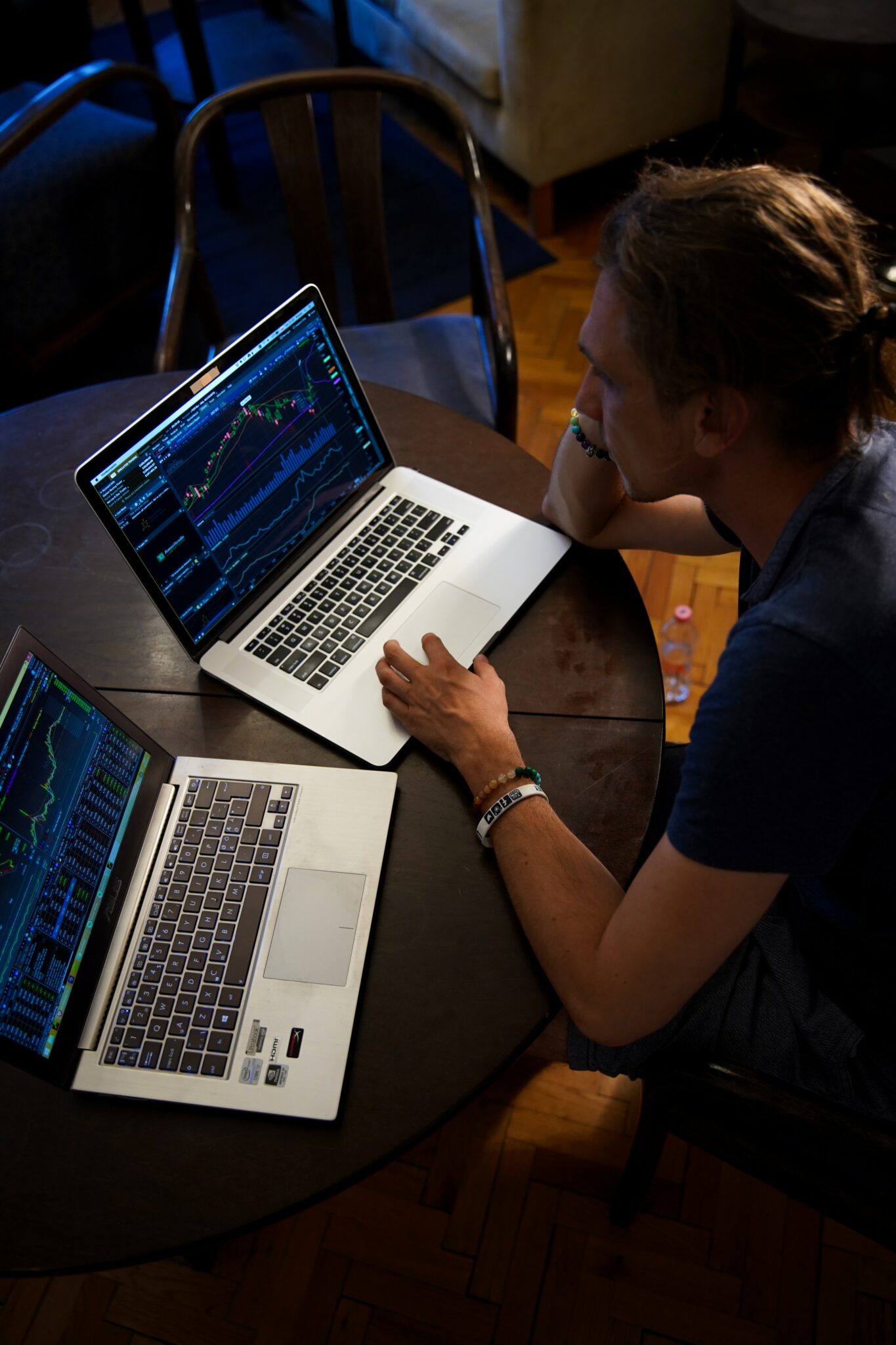
How to get started with Data Governance
Many of our customers have grown primarily through mergers and acquisitions to achieve accelerated growth.
As a result, the organization quickly faces the prospects of multiple applications and systems that deliver similar functions and store the same data. Ultimately, this can prevent an enterprise from gaining a holistic view of their organization.
As these businesses experience rapid and continuous growth, consolidating or completely integrating all these applications is a massive undertaking or, in some cases, an afterthought. Due to these fragmented systems, varying business processes and methods create many duplicate and silo versions of the organization’s truth. For example, how can an enterprise quickly achieve a single 360-degree view of the customer or a customer master dataset with multiple data sources managing and storing this data?
Different versions of the truth make it extremely difficult for any organization to deliver reporting and analytics capabilities that support fact-based decisions and policy consistently. Managing and preventing issues require valuable resource time, and the challenges often compound over time. These problems typically become noticeable when the organization faces more pressure to grow rapidly or when cost savings become a focus.
Such complications result from the business not recognizing the value and benefit that an enterprise and well-managed data governance framework and program brings to an organization and plays in the execution of roles, responsibilities, tasks, and decision making. The consequence represents the lack of:
- Formal strategy for data governance framework
- Executive sponsorship and support
- Data ownership from the business
- Resources and tools
- Budget
- Documentation
Our strategy is to get organizations started with a crawl, walk, and run approach. We like to prove the concepts and gain support from stakeholders and the right people with a production pilot project that solves a small, high-value use case. We demonstrate our capabilities while delivering a successful data application we can showcase to the business. This pilot program shows how data governance supports the quality and scalability of technology and data applications and tools and makes it much easier to gain executive sponsorship, allowing for the program’s advancement.
Organizations must utilize a holistic approach with the business process that collects and manages their valuable data in order to stay compliant, reduce costs and mitigate risks. Although it will undoubtedly come with its challenges, the successes of an organization’s program in the earlier stages can be reapplied on a larger scale as the organization and its operations mature. This minimizes the risk of failure while leveraging the strategies and mechanisms that brought positive changes and benefits in the past. A result is an evolutionary approach to data governance that we believe is the best chance for a substantial enterprise-wide data management initiative.
At Data Ideology, our experts understand the challenges associated with implementing an enterprise data governance program and its best practices across the company. Our expert consultants work to engage the business and gain support from executive leadership and stakeholders, which is crucial to a data governance program’s success. This allows us to deliver value and enable better business outcomes by integrating data governance into any data related process.